In 1974, some inspirational scientists, at what was then International Paints (now AkzoNobel’s Marine Coatings Business), set up a database to collect information on the performance of the company’s fouling control coatings. The database, called Dataplan, was designed with simplicity in mind, and grew unabated for almost 30 years.
Dataplan ultimately grew into “big data”; the world’s largest dataset on the performance of antifouling coatings on in-service vessels. In early 2002 this came to the attention of myself and David Williams, now R&D Director, Marine Coatings at AkzoNobel. We applied for and won a Royal Society Industrial Fellowship, which ran from 2003 to 2007.
The aim of the Royal Society project was to analyse Dataplan and to produce a model that could predict the performance of an in-service coating that was applicable to all coatings, all vessels, operating anywhere in the world: a truly global model. This was ambitious because the size of Dataplan pushed the then current PC technology to its limits.
The project resulted in a model that included vessel type, coating type, year of coating application, vessel size and speed, and area of vessel coated; this predicted successful coating performance. We used this model to show how the performance of the coatings depends not just on its formulation, but also many other factors including ship type, speed, and size etc. We were also able to compare the relative performance between the different technologies. For example, one clear conclusion was that the then new foul release and advanced self-polishing copolymer (SPC) were just as good as the older tin SPC (TBT) formulations.
It is now ten years since we did the final analysis and Dataplan has continued accruing data, and at the same time our skills have also grown. Time to revisit the old analysis!
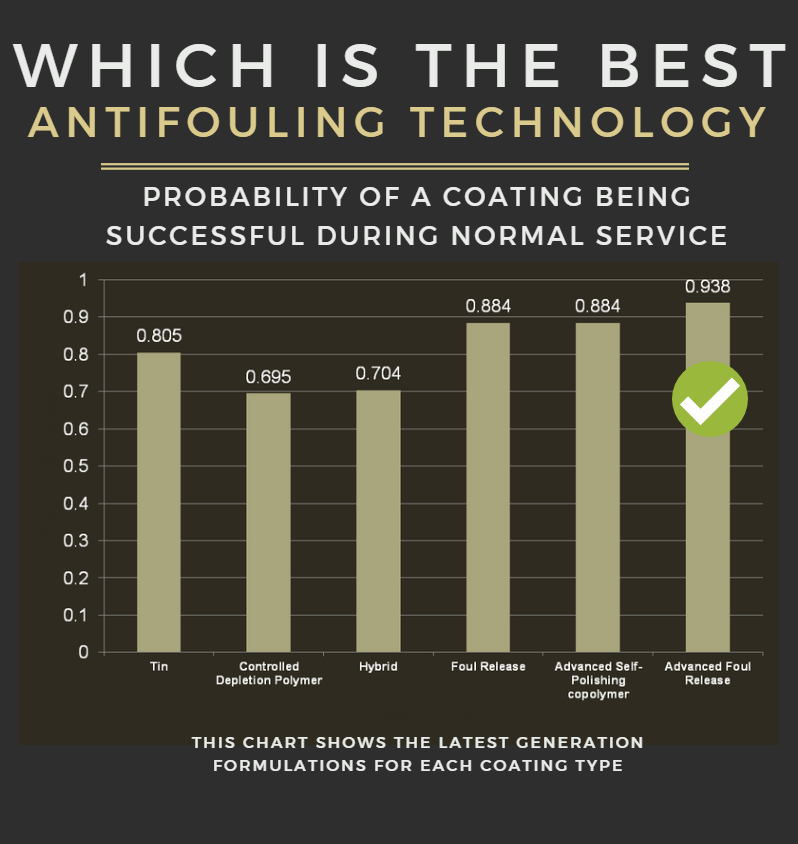
The first task was to run the data through the model we had originally devised during the Royal Society project and test it against a broader range of models available to us now.
We found that the original model was not out-of-date and when compared with the most appropriate new model available it could predict correctly the performance of a coating 75% of the time. This is a good result given the global nature of the data, the variety of operational profiles, the breadth of coating types and in-service intervals the coatings are used for. The model discriminates well between the coating technologies and shows how the foul release and advanced SPC products are outstanding with the advanced foul release coatings having a probability of performance success of 0.94 with the lowest performing coatings, the controlled depletion polymer (CDP), probability of performance success of 0.70. Thus we may consider the foul release coatings to be about 1.34 times better than the CDPs.
Perhaps the best outcome of this work is that all high performance fouling control systems coating technologies perform better than the old tin SPC products. There was concern that when the IMO ban on TBT formulations was proposed that non-TBT products would not perform as well as their replacements and that there would be an associated increase in greenhouse gas emissions and clearly this is not the case.
Since we revisited the old data analysis, we have focussed on building models to predict the actual amount of fouling on in-service coatings, not just whether they are likely to succeed or not. This proved to be a much harder task and after several years’ work pushing the data through ever more esoteric algorithms we realised that we needed more data to explain the variation in coating performance that we were seeing. A significant hole in the data was the lack of voyage information for each vessel; however with the global adoption of AIS we now have access to the position and speed of each and every vessel from 2009 onwards. Combining this with Dataplan and a suite of environmental data has created a true “big data” database, with 1,000s of gigabytes of data, compared to 10s of gigabytes, and this requires expertise not only in mathematics, but also systems engineering, geospatial analysis as well as in machine learning to pull it all together. This was the approach of the InnovateUK project, HELM, led by Richie Ramsden at AkzoNobel’s Marine Coatings Business, based in the UK. In HELM we moved the analysis from the desktop to the cloud, from focussed hypothesis driven-modelling to machine leaning analytics running inside the database. Along the way we have provided insight into comparative product performance, insight into key variables affecting this performance, and delivered new data-based products: Intertrac, Intertrac Vision, with more to come in the pipeline. Data analytics have arrived after more than 40 years of truly inspired data collection.
@ Jeremy C Thomason, Exoteknica SCP, Merida, Mexico
Contact us
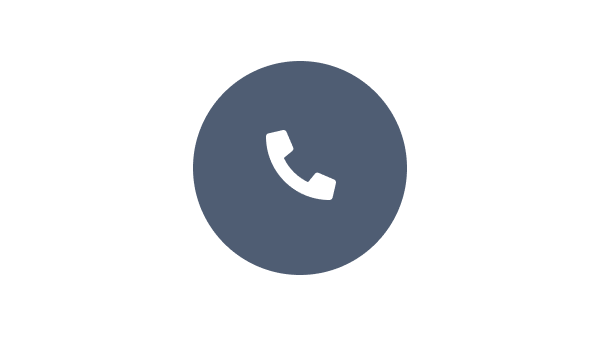
We are here to help
Speak with one of our experts to discuss how we can get the best results for your area of application.
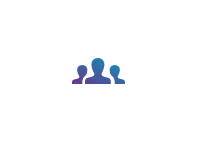
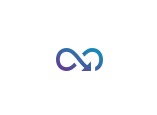
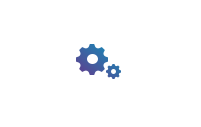